Keynote Speakers
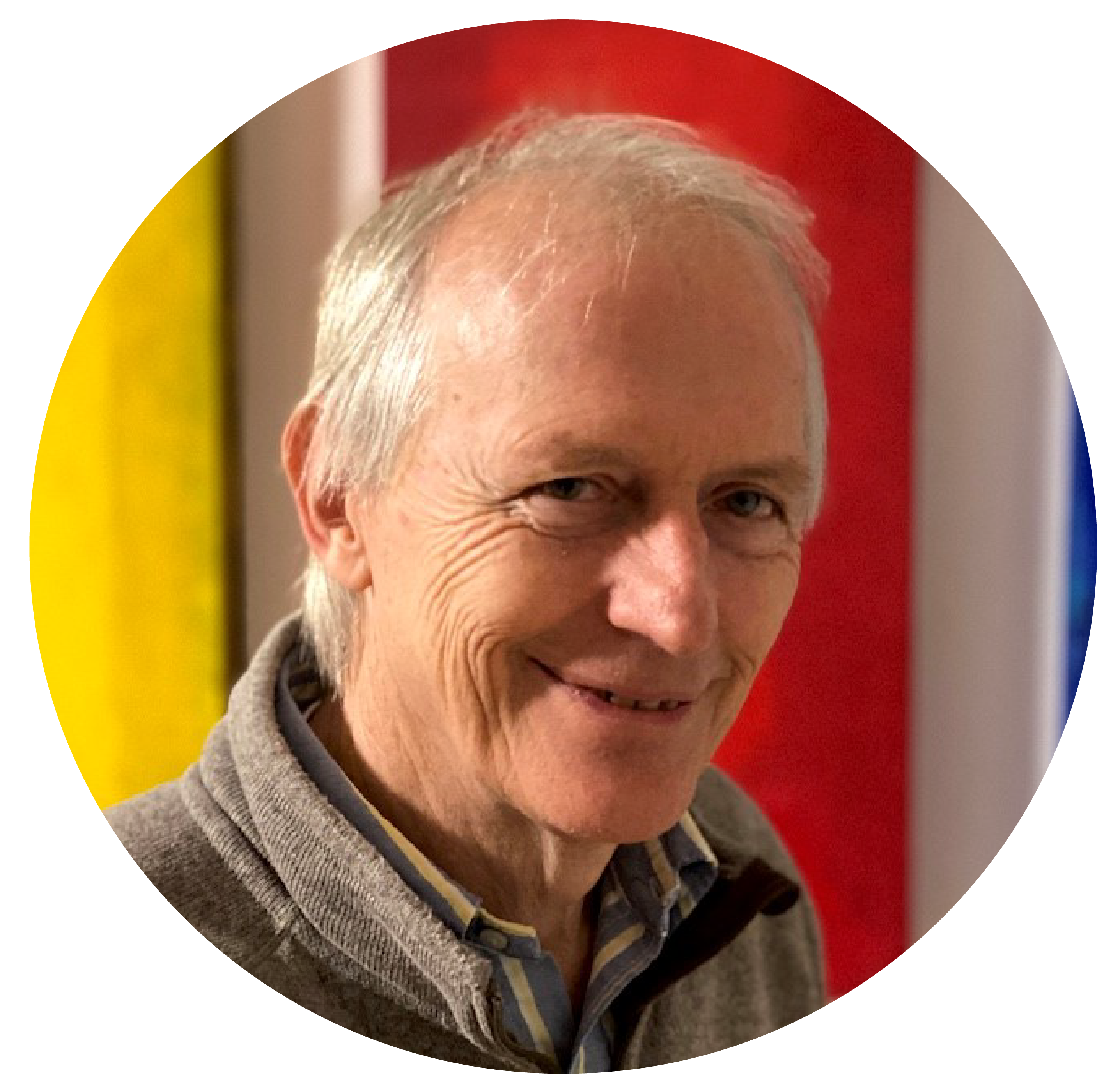
Color vision for objects
Karl R Gegenfurtner
Professor in Psychology at Giessen University, Germany
Bio
Karl Gegenfurtner studied Psychology at Regensburg University. Subsequently he obtained a Ph.D. degree from New York University, where he also spent his first PostDoc. In 1993 he moved to the Max-Planck-Institute for biological cybernetics in Tübingen, where he obtained his Habilitation in 1998 and a Heisenberg-Fellowship in the same year. In 2000 he moved to the University of Magdeburg and in 2001 to Giessen University, where he since then holds a full professorship for Psychology. The emphasis of Karl Gegenfurtner’s research is on information processing in the visual system. Specifically, he is concerned with the relationship between low level sensory processes, higher level visual cognition, and sensorimotor integration.
Karl Gegenfurtner is the head of the DFG Collaborative Research Center TRR 135 on the “Cardinal mechanisms of perception”. He was elected into the National Academy of Science Leopoldina in 2015, received the Wilhelm-Wundt medal of the German Psychological Association (DGPS) in 2016 and an ERC Advanced Grant on color vision in 2020.
Abstract for presentation
Color vision for objects
The study of color vision in humans has been a successful enterprise for more than 100 years. In particular, the establishment of colorimetry by the CIE in 1931 has brought forward tremendous advances in the study of color in business, science, and industry (Judd 1952). During the past 50 years, the processing of color information at the first stages of the visual system—in the cone photoreceptors and retinal ganglion cells—has been detailed at unprecedented levels of accuracy. Has color vision been solved? I will argue that a transition from flat, matte surfaces to the color distributions that characterize real-world, 3D objects in natural environments is necessary to fully understand human color vision. I will present results from Virtual Reality psychophysics and from Deep Neural Network modeling that show the importance of objects for color discrimination, color constancy and the emergence of color categories.
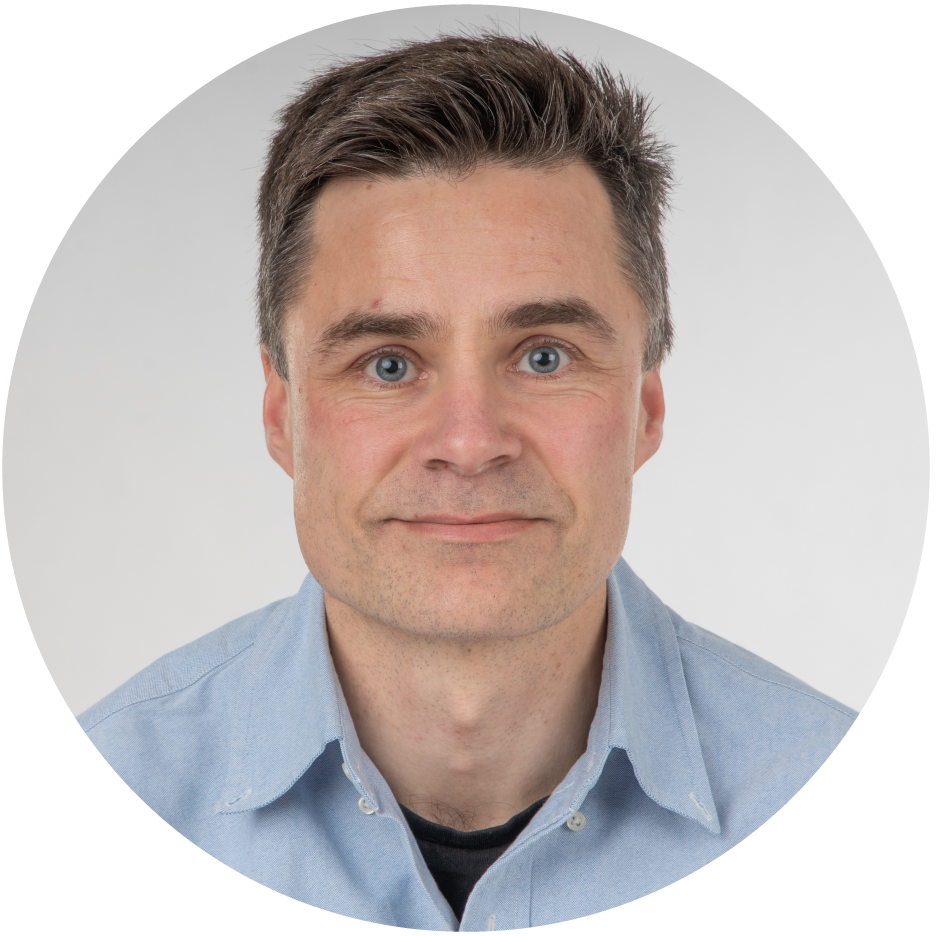
Visual Intelligence for medical image analysis
Robert Jenssen
Director of SFI Visual Intelligence and professor at UiT The Arctic University of Norway
Bio
Robert Jenssen directs Visual Intelligence, a centre for research-based innovation (SFI) funded by the Research Council of Norway and consortium partners. Jenssen is a Professor in the Machine Learning Group at UiT The Arctic University of Norway. He is also an Adjunct Professor at the University of Copenhagen, Denmark, and at the Norwegian Computing Center in Oslo, Norway. In his research, Jenssen is focusing on advancing deep learning and machine learning methodology for complex image analysis and data analysis in general. He collaborates with industry and at the intersection of technology and health. Jenssen received the Dr. Scient (PhD) degree from UiT in 2005. He has had long-term research stays at the University of Florida, at the Technical University of Berlin, and at the Technical University of Denmark. Jenssen has international leadership experience, e.g. as a member of the IEEE Technical Committee on Machine Learning for Signal Processing, as a member of the Governing Board of IAPR, and an Associate Editor for the journal Pattern Recognition. He has been the leader of the Norwegian association on image analysis and pattern recognition. He was the general chair of the Scandinavian conference on image analysis, 2017. He is a general chair of the annual Northern Lights Deep Learning Conference, NLDL.
[@jenssen_robert / visual-intelligence.no / machine-learning.uit.no / nldl.org]
Abstract for presentation
Visual Intelligence for medical image analysis
In deep learning for medical image analysis, exploitation of limited data, in the sense of having few annotations, is a key challenge. Transparency is also a challenge, in the sense of revealing biases, artefacts, or confounding factors, on the path towards more trustworthy analysis systems. This talk outlines some lines of research in Visual Intelligence to tackle these challenges. The first part of the talk focuses on medical image segmentation when little labelled data is available by developing an anomaly detection-inspired approach to few-shot learning. The second part focuses on XAI (explainable AI) by developing a self-explainable model to highlight potential challenges obtained when leveraging several different image data sources for diagnostics as well as to reveal causes in the form of image artefacts.
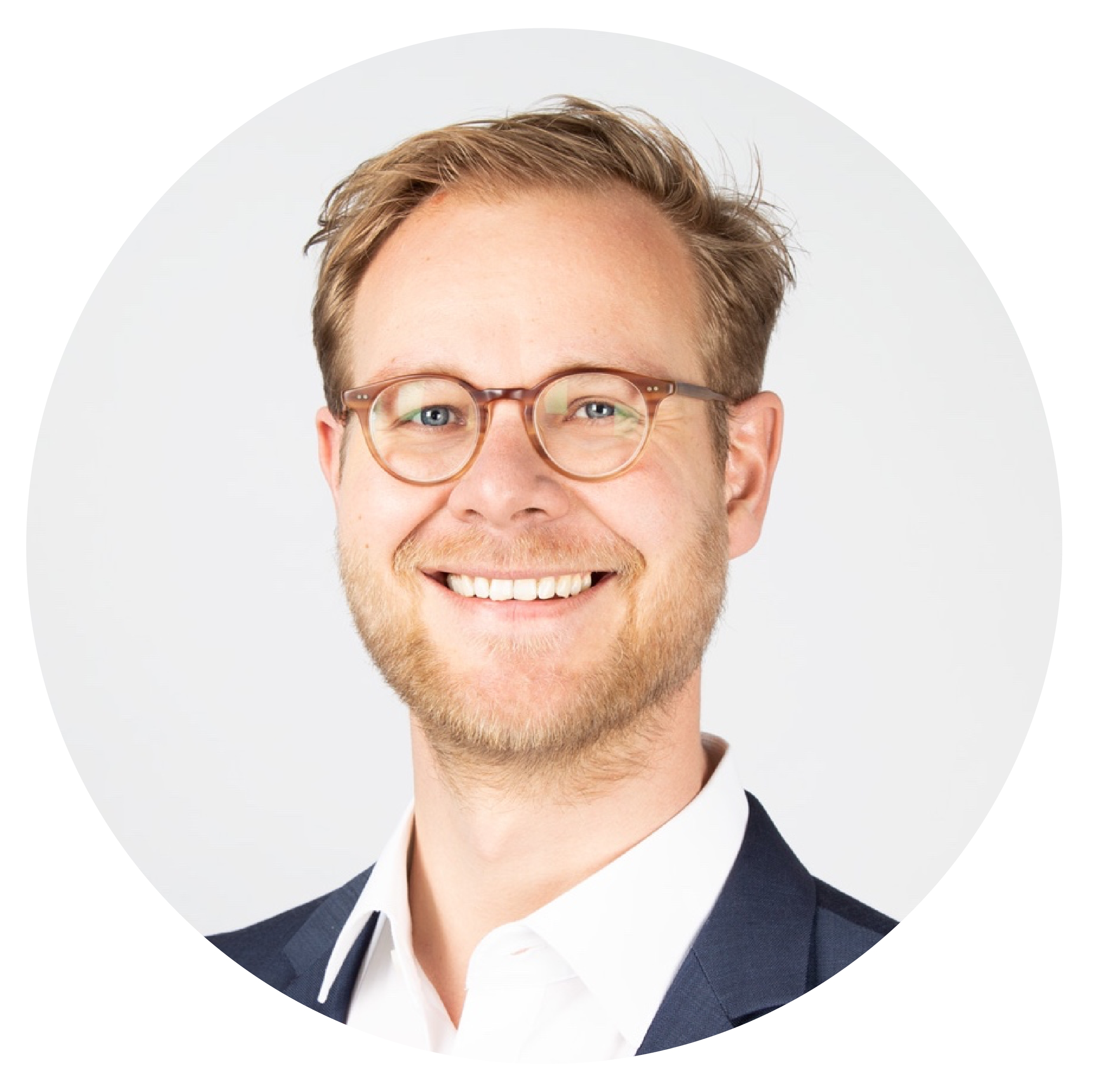
Neural approaches to visual quality estimation
Sebastian Bosse
Head of Interactive & Cognitive Systems Group at Fraunhofer HHI – Heinrich Hertz Institute
Bio
Sebastian Bosse is head of the Interactive & Cognitive Systems group at Fraunhofer Heinrich Hertz Institute (HHI), Berlin, Germany. He studied electrical engineering and information technology at RWTH Aachen University, Germany, and Polytechnic University of Catalonia, Barcelona, Spain. Sebastian received the Dr.-Ing. degree in computer science (with highest distinction) from the Technical University Berlin in 2018.
During his studies he was a visiting researcher at Siemens Corporate Research, Princeton, USA. In 2014, Sebastian was a guest scientist in the Stanford Vision and Neuro-Development Lab (SVNDL) at Stanford University, USA.
After working as a research engineer in the Image & Video Compression group and later in the Machine Learning group, he founded the research group on Interactive & Cognitive Systems at Fraunhofer HHI in 2020 that he has headed since.
Sebastian is a lecturer at the German University in Cairo. He is on the board ort h Video Quality Expert Group (VQEG) and on the advisory board ort h Interational AIQT Foundation. Sebastian is an affiliate member of VISTA, York University, Toronto, and serves as an associate editor for the IEEE Transactions on Image Processing.
His current research interests include the modelling of perception and cognition, machine learning, computer vision, and human-machine interaction.
Abstract for presentation
Neural approaches to visual quality estimation
Accurate computational estimation of visual quality as it is perceived by humans is crucial for any visual communication or computing system that has humans as the ultimate receivers. But most importantly besides the practical importance, there is a certain fascination to it: While it is so easy, almost effortless, to assess the visual quality of an image or a video, it is astonishingly difficult to predict it computationally. Consequently, the problem of quality estimation touches on a wide range of disciplines like engineering, psychology, neuroscience, statistics, computer vision, and, since a couple of years now, on machine learning. In this talk, Bosse gives an overview of recent advances in neural network-based-approaches to perceptual quality prediction. He examines and compares different concepts of quality prediction with a special focus on the feature extraction and representation. Through this, Bosse revises the underlying principles and assumptions, the algorithmic details and some quantitative results. Based on a survey of the limitations of the state of the art, Bosse discusses challenges, novel approaches and promising future research directions that might pave the way towards a general representation of visual quality.
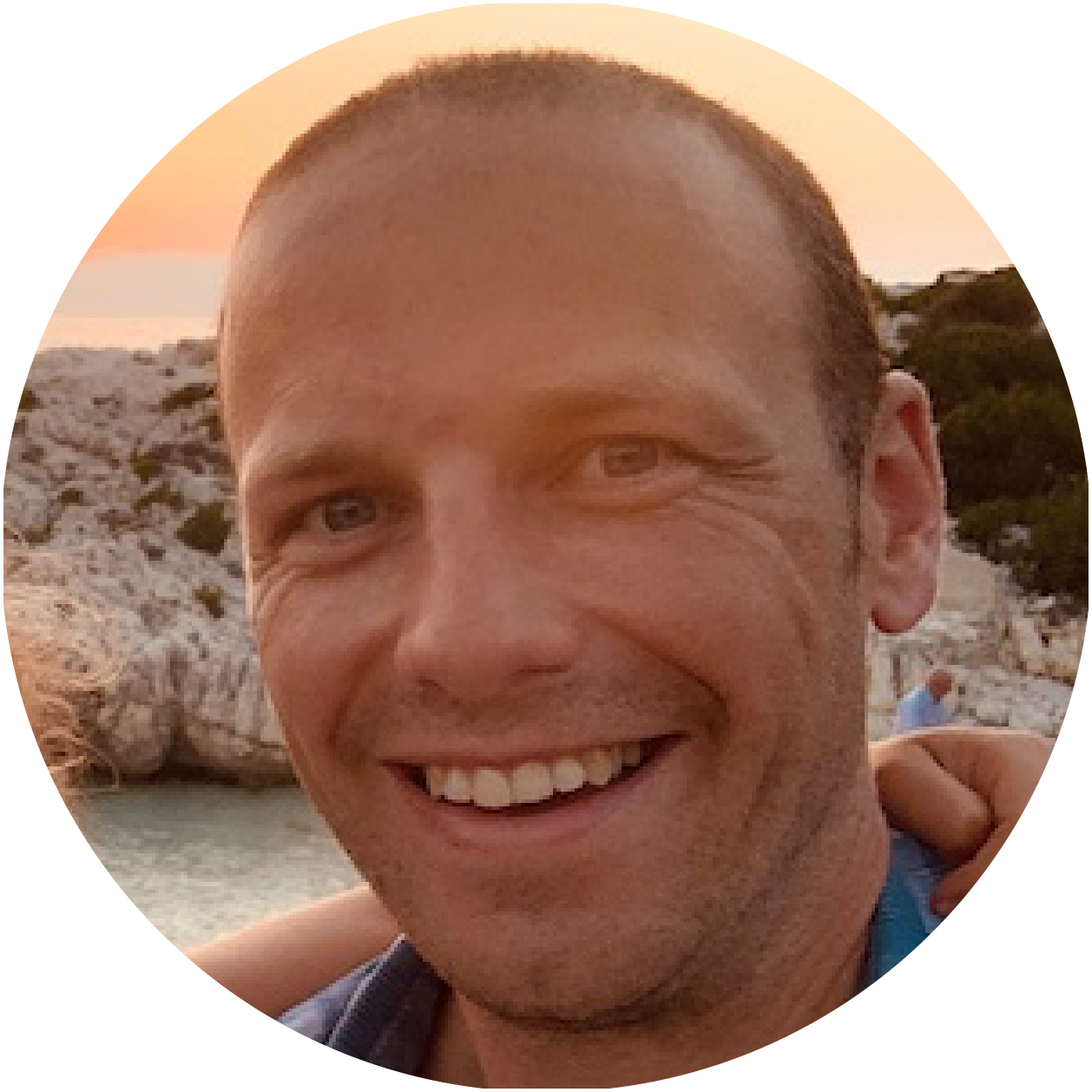
Self-supervised Inverse Rendering
William Smith
Reader in Computer Vision, Department of Computer Science, University of York, UK
Bio
Will Smith is a Reader in the Department of Computer Science, University of York where he leads the Vision, Graphics and Learning (VGL) research group. He was a Royal Academy of Engineering/The Leverhulme Trust Senior Research Fellow from 2019-2020 and is currently an Associate Editor of the journal Pattern Recognition. He currently leads a team of two postdocs and ten PhD students and has published over 100 papers, many in the top conferences and journals in the field. His research interests span vision, graphics and ML. Specifically, physics-based and 3D computer vision, shape and appearance modelling and the application of statistics and machine learning to these areas.
Abstract for presentation
Self-supervised Inverse Rendering
Inverse rendering is the task of decomposing one or more images into geometry, illumination and reflectance such that these quantities would recreate the original image when rendered. Deep learning has shown great promise for solving components of this task in unconstrained situations. However, the challenge is a lack of ground truth labels to use for supervision. Will Smith will describe a line of work that learns to solve this problem for outdoor scenes with no ground truth. They are based on extracting a self-supervision signal from unstructured image collections alone while introducing model-based constraints to resolve ambiguities. He will describe both single image methods, that learn general principles of inverse rendering, and multi-image methods that fit to a single scene by extending Neural Radiance Fields to relightable outdoor scenes. Smith will describe priors that we enforce on natural illumination and results on the application of photorealistic scene relighting.